Predictions
Prediction is an information output generated by an ML algorithm trained on historical input data.
What is a Prediction?
A machine learning prediction is simply a model’s output when provided with an input example containing one or more features and a label.
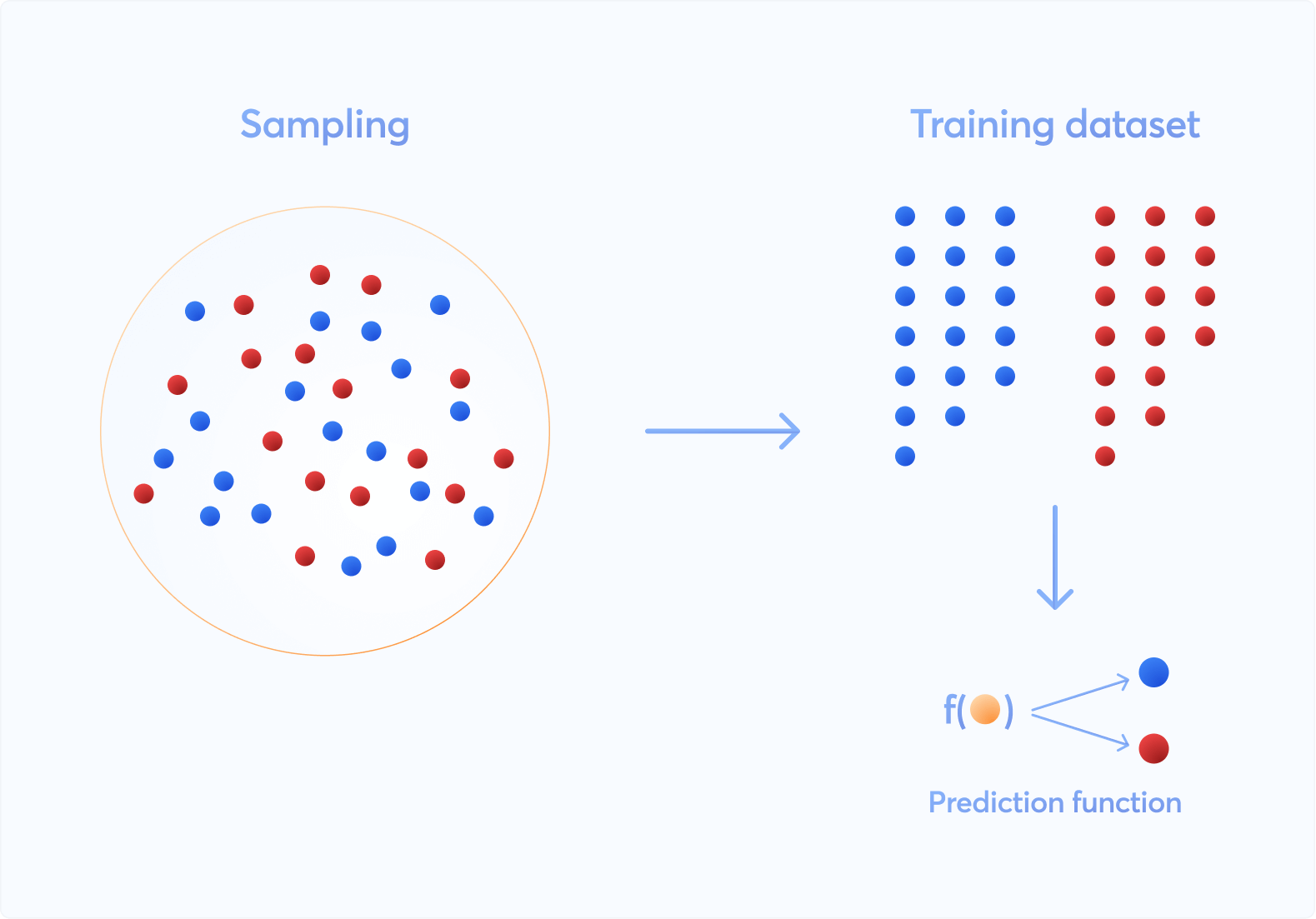
The word prediction is not essentially connected to future actions, but it sometimes refers to making a guided guess on past events. For example, predicting a specific transaction was legitimate or fraudulent requires analyzing a past event - a transaction that has already taken place. However, deciding the best marketing strategy to avoid customer attrition requires predicting the likelihood of customer churn.
Why does Accurate Prediction Matter?
Reliable ML predictions offer valuable insights leading to more confident and guided decisions by businesses. Different examples include but are not limited to
- Business sales forecast for the next quarter
- Likelihood of customer churn for a specific brand
- The best schedule for the predictive maintenance of equipment
- Credit default prediction
- Prediction for real-time and personalized recommendations
Key Challenges in Making Predictions
ML-based prediction systems collect training data from appropriate resources, such as health records, public resources like map or weather information, or geolocation data captured by devices and sensors. The real challenge comes with the unstructuredness and the quality of data captured through these various resources.
Another key challenge includes the requirement of periodically and systematically retraining models. Data drift, concept drift, model staleness, and data skew hamper the quality of ML predictions making model retraining a must.
Predictive modeling is also challenged by the efficiency of feedback loops to learn from outcomes and improve the quality of the following prediction cycle. Negative feedback loops that propagate biased outcomes and inaccurate results constitute a true challenge.
AI Observability - A Key to Accurate ML Predictions
“The only constant in the ML world is the change itself.”
ML models tend to deviate in their results from the expected distribution over time. Predictions need to be refined with data provided to production ML models. But this data itself changes for several reasons like data drift, target change, or new concept introduction. We can not control changes in datasets, but we can observe the datastream for changes.
The introduction of Observability in ML systems ensures models are performant with acceptable accuracy. This also helps determine the best time to retrain your models.
In simple cases monitoring standard performance measures like F1-score, accuracy, ROC AUC is sufficient. For more complicated cases, confirming the new set of predictions has a similar distribution to the training data is recommended.
Monitoring these metrics becomes impossible if you rely on manual options. However, modern AI Observability solutions such as Censius AI Observability Platform automate monitoring. It accelerates your ML ventures with proactive steps backed by a prompt alerting system.
Further Reading
Monitor! Stop Being A Blind Data-Scientist.
Monitoring Machine Learning models
Monitoring Machine Learning Models in Production