Bias In Machine Learning
ML bias is the effect of erroneous assumptions used in the processes or prejudices in the data producing systematically prejudiced results
What is Bias in ML?
Machine learning bias is also called AI bias or algorithm bias. It is the effect of erroneous assumptions used in the machine learning process or prejudices in the training data producing systematically prejudiced results.
Machine learning bias is observed in many forms - gender prejudice, racial bias, age discrimination, and recruiting inequality.
ML solutions show cognitive bias due to human prejudice – conscious or unconscious. Here cognitive bias reflects feelings towards a person or subpopulation based on their perceived group membership. There are 180+ types of cognitive biases defined and classified by psychologists that affect ML solution's performance. For example, bandwagon effect, selective perception, priming, and confirmation bias.
Another common reason to induce ML bias is the lack of complete data. The training data used to train models is not complete or may not be a true representative of ground truth. Low-quality training data sometimes reflects societal or historical inequalities causing AI bias. Faulty, incomplete datasets result in inaccurate predictions delivered by ML systems, causing garbage in garbage out.
Examples of ML Bias
Amazon's Recruiting Tool
Amazon introduced an AI-powered tool to automate resume screening for recruitment processes. However, the tool preferred men over women. It was not rating candidates for software development and other technical jobs in a gender-neutral way.
Racial bias by the healthcare risk model
A healthcare risk prediction algorithm showed a racial bias for a specific subpopulation. The model relied upon faulty metrics to determine the risk. The algorithm favored white patients over dark-skinned patients to predict patient names for extra care.
Types of Machine Learning Bias
Sample bias: Sample bias occurs when the data used is not the right representative to train the model
Prejudice bias: The data used to train the ML model demonstrates existing prejudices, stereotypes, or faulty societal assumptions leading to ML systems with real-world bias
Measurement bias: This type of bias is introduced due to underlying issues with the accuracy of the data and how it was computed
Exclusion bias: Exclusion bias occurs when a critical data point is missed or leftover from the training dataset
Algorithm bias: Algorithm bias occurs due to a problem within the algorithm used to deliver predictions by ML models.

Fixing bias in ML systems
Mckinsey suggests the following practices to fix and mitigate ML bias:
- Fully understand algorithms and data to evaluate the risk of bias for ML systems
- Establish processes and practices to mitigate bias in ML systems. Focus on technical, operational, and organizational debiasing strategies
- Understand potential biases in human decisions
- A balance between automated decisions and manual interventions,with a preferred choice of manual interventions in sensitive issues
- Implement a multidisciplinary approach with a focus on bias research and data collection
- Diversified AI teams to help mitigate ML biases
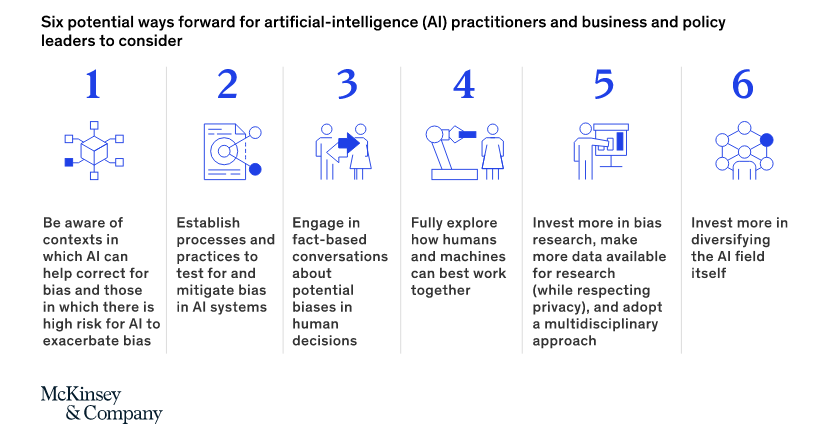
Using different tools and libraries to detect and mitigate machine learning bias is beneficial. These tools help test biases in ML models and datasets using a good set of metrics. Examples include IBM’s AI Fairness 360, IBM Watson OpenScale, and Google’s What-If tool.
Further Reading
Amazon scraps secret AI recruiting tool that showed bias against women
Bias in AI: What it is, Types, Examples & 6 Ways to Fix it in 2022