Model Monitoring
ML monitoring is the practice of tracking the performance of ML models in production to identify potential issues in ML pipelines
What Is Model Monitoring?
ML model monitoring is the practice of tracking the performance of ML models in production to identify potential issues that can add negative business value. These practices help proactively monitor prediction quality issues, data relevance, model accuracy, and bias.
ML monitoring constitutes the subset of AI observability where it showcases a bigger picture with testing, validation, explainability, and exploring unforeseen failure modes.
The performance of ML models starts degrading over time. It can be due to data inconsistencies, skews, and drifts, making deployed models inaccurate and irrelevant. Appropriate ML monitoring helps identify precisely when the model performance started diminishing. Such proactive monitoring helps take required actions like retraining models or replacing models. It helps foster users’ trust in ML systems.
Why is ML Model Monitoring Important?
ML monitoring helps fix the issue of poor generalization that arises due to training models on smaller subsets of data. With poor generalization, ML models fail to attain the required accuracy. Changes in data distributions over time also affect model performance.
A model is trained and optimized based on the variables and parameters provided to it. These same parameters may fail to hold ground truth or become insignificant when the model is deployed. Monitoring practices help analyze how the deployed model performs on real-time data over a long period.
ML models involve complex pipelines and automated workflows. Without an appropriate monitoring framework, these transformations become error-prone and hamper the model’s performance over time.
Inputs fed to ML models can destabilize ML systems due to changes in sampling methods or hyper-parameter configuration changes. Tracking different stability metrics helps stabilize the ML system.
ML model monitoring helps understand and debug production models and the derived insights help reduce complications raised due to the black-box nature of ML models.
How Censius Helps?
ML monitoring helps for sure. Now the key question is – how to do that? It’s a breeze with advanced monitoring solutions like Censius AI Observability Platform. The platform helps automate tracking of production ML model performance and the entire pipeline.
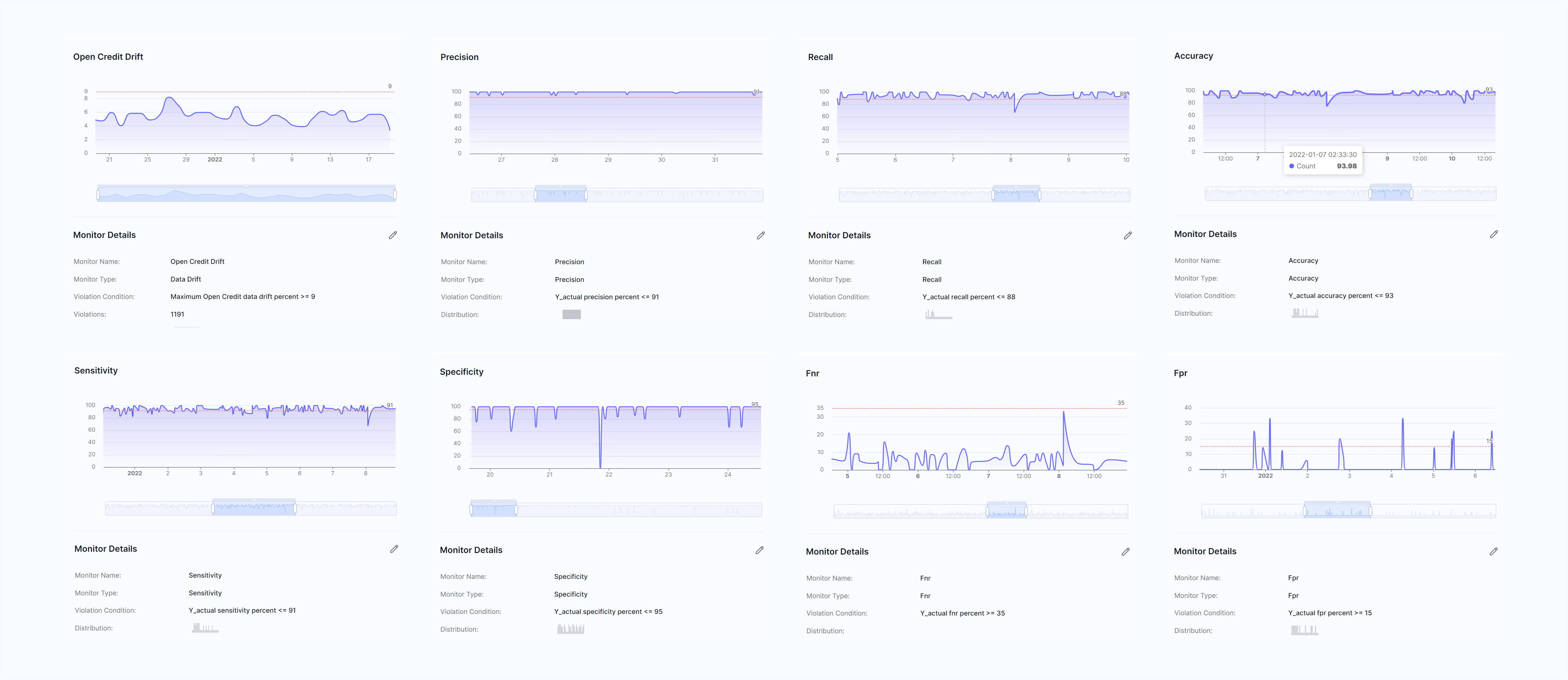
Censius enables setting and customizing ML monitoring solutions for
- Data Quality Monitors: Monitor data quality issues like missing data, new value, data range, and data type mismatch
- Drift Monitors: Data and concept drift monitors
- Model activity monitors: Track the number of predictions the model serves over time
- Performance monitors: Track performance metrics like precision, recall, F1 score, sensitivity, specificity, FNR, and FPR.
Censius monitoring platform automates the tracking of ML model performance and helps your teams to focus on more pressing issues.
Further Reading
Best Tools to Do ML Model Monitoring