AI Observability
AI observability is a holistic and complete approach to drive insights on the model’s behavior, data, and performance across its lifecycle.
What is AI Observability?
AI observability is a modern, holistic and complete approach to drive insights on the ML model’s behavior, data, and performance across its lifecycle. It enables precise root cause analysis of predictions made by ML models and helps build performant and responsible models. AI Observability brings a proactive approach to detect ML pipelines issues and take necessary actions to avoid more losses. It helps foster people’s trust in ML systems.
Although ML monitoring and observability seem similar, observability denotes a bigger picture with testing, validation, explainability, and preparedness for unpredictable failure modes.
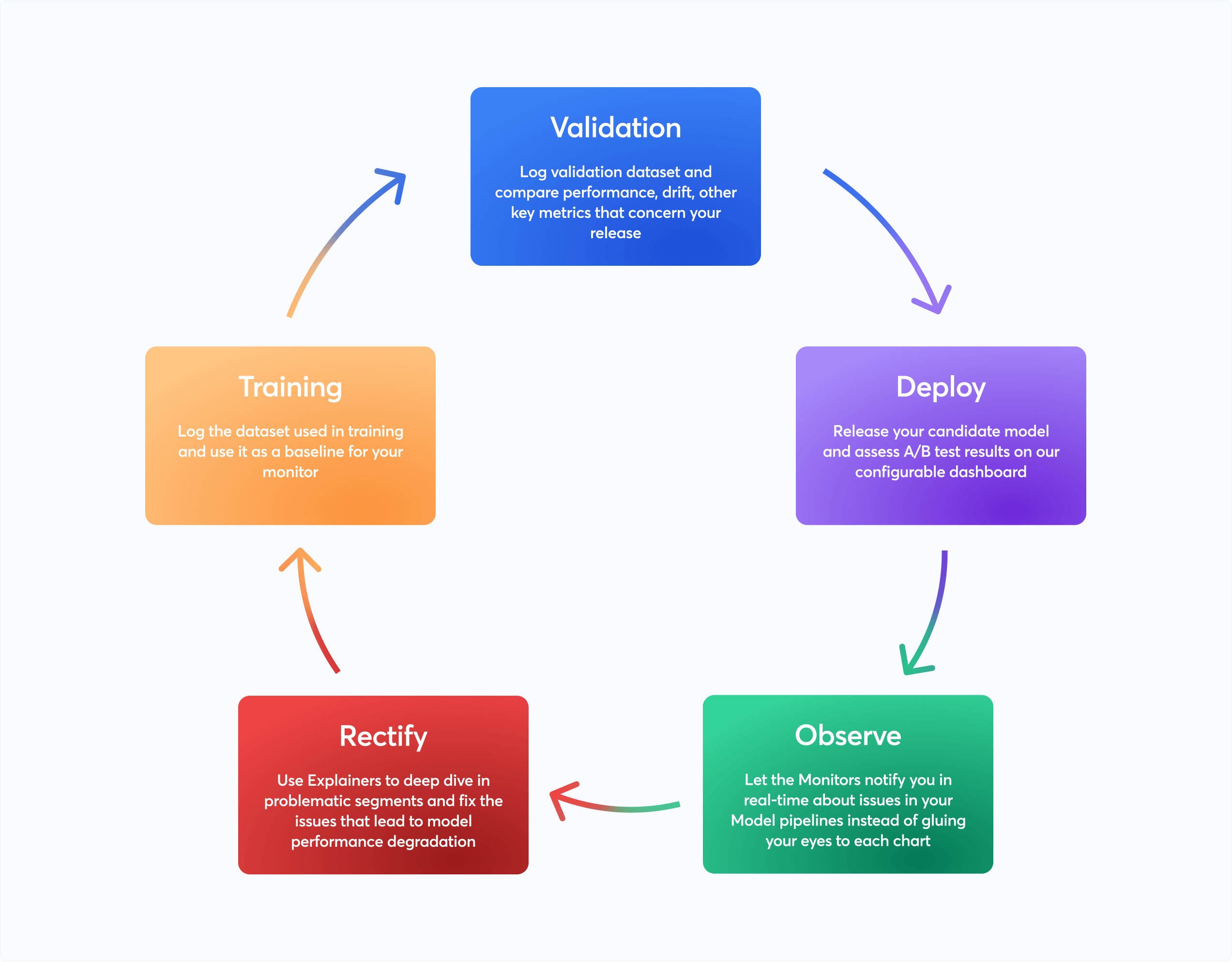
Why is AI Observability Essential?
AI observability helps drive deep insights about ML models and entire pipelines. It provides visibility into the model functioning and helps make decisions on models such as:
- Deployment of a new candidate model under an A/B test
- Replacement of an ML model with a new model
- Minor tweaks to the live and operational model
Machine learning observability helps obtain insights on model performance, data quality issues, model degradation, and model behavior. It helps address ML systems with:
Data skew: Data skew is observed when model training data fail to represent the live data. Skew occurs due to many reasons such as mismatch in training and production data, data dependencies, faulty design of training dataset, upstream data changes, and data integrity issues.
Model staleness: Different factors such as shifts in the digital environment, economic conditions, changes in consumer preferences, and foreign governments contribute to ML model decay over time if you are not updating your models.
Inappropriate feedback systems: ML systems face severe issues with biased, inaccurate, or corrupted data, and these inputs are propagated in further prediction cycles, resulting in poor quality outcomes.
AI Observability: A Holistic and Complete Approach
Advanced AI observability solutions such as the Censius AI Observability Platform facilitate automated monitoring of ML pipelines, data, and models. Its proactive approach in monitoring ML pipelines helps identify and fix issues quickly. It also simplifies monitoring thousands of ML models simultaneously.
The Censius AI Observability Platform promptly alerts on model anomalies helping your teams take further actions such as model retraining.
The platform serves any AI infrastructure to detect ML pipelines issues and performs root-cause analysis. It offers a comprehensive ML monitoring solution that includes:
- Recognizing drifts, data quality issues, and model activity
- Tracking specific model performance metrics
- Analyzing data segments for better insights
Further Reading
Machine Learning Observability and Monitoring
Monitoring Machine Learning Models in Production