AI Audit
AI audit defines a piloted audit program to educate C-suite leaders, expose risks involved, and accordingly develop safeguard controls
What is AI Audit?
AI auditing is a modern approach to educate the C-suite about the value of AI adoptions, uncover the risks involved to the businesses, and develop safeguard controls to avoid these risks.
While AI drives competitive advantage to enterprises, it also brings numerous potential risks and pitfalls. The explosion of big data requires appropriate controls to ensure the proper use of data generated every moment. Different global organizations have defined many AI auditing frameworks with a set of principles and guidelines to aid AI auditing. These frameworks help businesses in their digital transformation journey while delivering positive business value.
AI audit includes well-defined and piloted audit programs intended to assure risk assessment and a high level of governance. Auditability in the AI landscape describes
- Evaluation of algorithms, models, and data streams
- Analysis of operations, results, and even unexpected outcomes
- Technical and ethical aspects of AI systems to focus on results accuracy and adherence to principles such as equality and privacy
Enterprise AI Audit Checklist
AI audit is crucial to building sustainable AI systems by understanding the capabilities of AI and KPI’s related to AI success. Auditing offers a comprehensive guiding framework to C-level and other stakeholders.
Audit AI outcomes
Successful AI adoption requires clarity on business objectives and AI's potential to achieve those. Decision stakeholders should confirm that their AI strategy is aligned to achieve desired goals and justifies their AI expenditures.
Audit data sources
AI audit includes different data sources - internal, third-party, and public data sources. Understanding data sources used and ensuring the accuracy of data used for AI audit helps define a better auditing practice.
Clean data
AI audit checklist is incomplete without clean data pipelines across the AI lifecycle. Garbage in garbage out - contextual in AI context.
Data privacy audit
Data privacy has been a high-priority concern for a modern AI system. Data privacy consideration in AI audit accounts for the implementation of privacy standards, protection of consumer rights, and legal aspects around data usage and privacy.
Accuracy of AI systems
AI system accuracy is significantly affected by changes in algorithms and data. Biased datasets might decrease the accuracy of AI systems over time. Continuous monitoring, algorithm assessment, and checking possibilities of potential vulnerabilities constitute better AI practices for AI accuracy audit.
Security audit
AI systems are more prone to security attacks and damage by hackers. Although AI brings tremendous opportunities, it also poses the risk of susceptibility to security breaches and data compromise. AI systems backed by tight security controls and standards help overcome different security loopholes.
AI Auditing Simplified with Modern Frameworks
In this digital era, various global organizations have defined many AI auditing frameworks to aid AI auditing. Check the list of such frameworks that can help you audit AI-enabled initiatives.
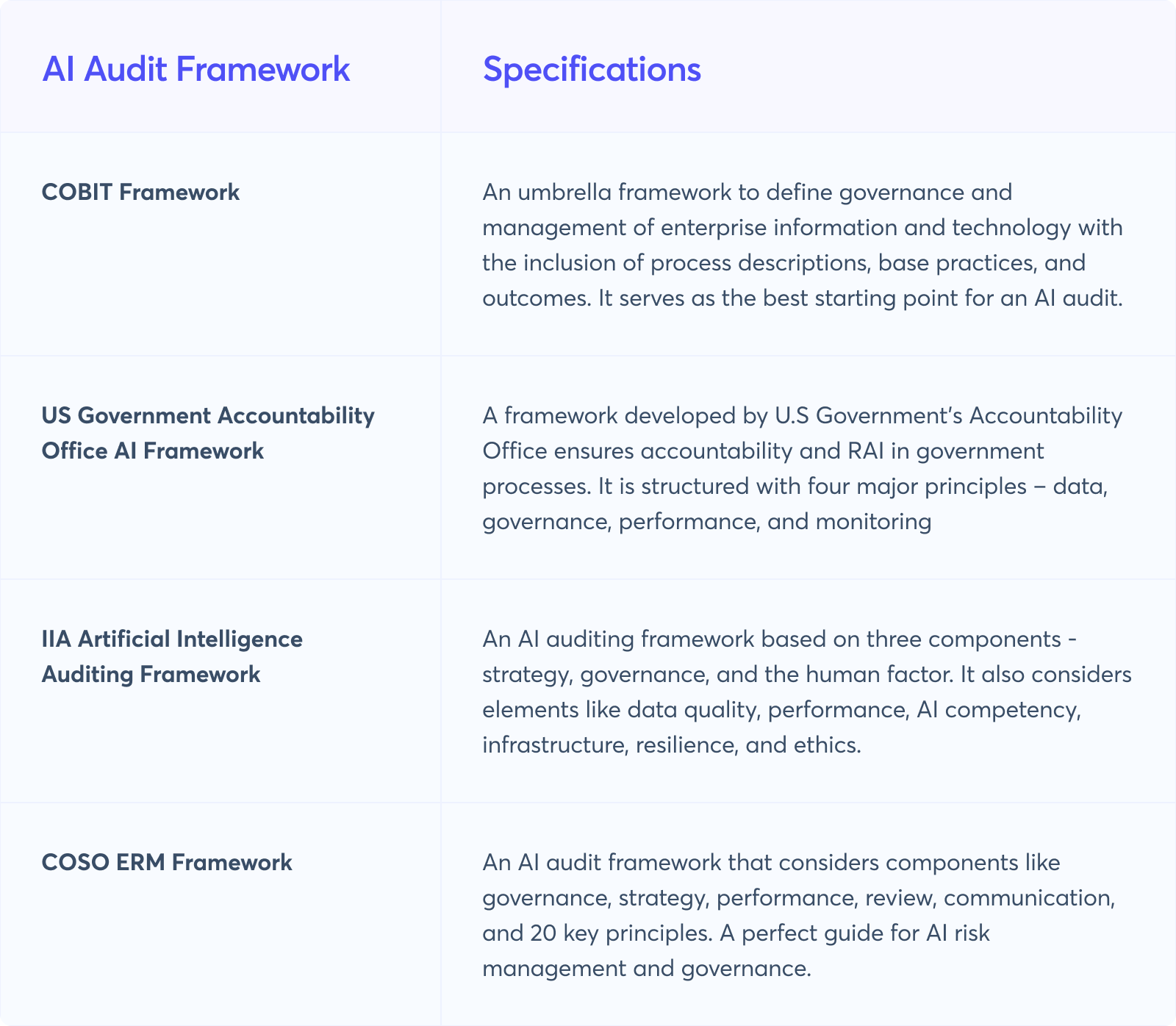
Further Reading
A Checklist To Audit Your AI Systems
Auditing Artificial Intelligence
Why AI must be included in audits
5 AI Auditing Frameworks to Encourage Accountability