This post will answer the following questions:
- What is trustworthy Artificial Intelligence (AI)?
- Is it the same as responsible AI?
- How can you achieve trustworthy AI?
-
Leveling Up from Trustworthy Computing
It was the time to put into action the holiday you had been planning for months. The plan kept getting canceled because of one friend or the other, but now it is go time! Together your group looked for the best accommodation. Someone saw an excellent recommendation on a website and decided to book it. Next came the flights and checking out things to do at the destination. Through all of this, computing systems made your plans come alive.
From browsing recommendations to the final boarding of the flight, you were well aware of the numerous computing systems which helped the plan. It was fun to check out all the possibilities a system fetched for you. It was also exciting to finally board the flight. Not for once did you doubt the airplane, which works with thousands of circuits and software because you know that they are well-tested.
If you think of airplanes and air traffic control systems, you associate them with reliability, safety, and well-tested security. This knowledge allows you to place trust in the ecosystem. Now, imagine that you had to sit in a self-driving car instead. Would you be as trusting with it?
Computing systems have been part of our lives for decades. Their gears have been well-worn, and many trust issues have been handled over time. But trust is still riddled with doubt t when it comes to AI systems. Besides the three qualities of reliability, safety, and security, AI requires further assurances.
- The first requirement is accuracy especially concerning unforeseen data.
- The second requirement is the sensitivity of the output toward input changes.
- The third requirement is ethics which encompasses other qualities like fairness, transparency, accountability, and privacy.
- The fourth requirement is relatively new and demands explainability of decisions and straightforward interpretations.
As an AI practitioner, you must prioritize accuracy, but sometimes a gold standard must be compromised for fairness. For instance, a loan credibility calculator must consider fairness towards gender and race over biased accuracy. Therefore, as shown in the image below, a curve can be used to ensure maximum accuracy is achieved for minimum bias.
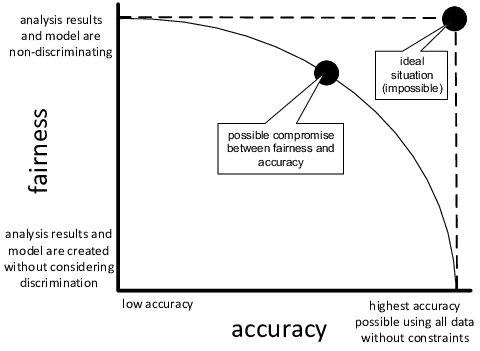
While conventional computing was all about discrete logic, AI is influenced by the human factor. The probabilistic AI decisions are based on the training-testing data and human perceptions. Additionally, deep learning added certain opaqueness. Therefore, our attention is directed to the trustworthiness of AI systems and how to address the tradeoffs and uncertainty. The trustworthiness of an AI system encompasses:
- To enable human agency and not impact their fundamental rights
- To ensure robustness against inconsistencies and security of AI algorithms
- To ensure data governance and privacy of individuals
- To provide transparency regarding decisions
- To enable fairness across race, gender, abilities, and socio-economic backgrounds
- To foster environmental responsibility and sustainability
- To provide accountability for the outcomes

Is Trustworthy AI Same as Responsible AI?
Literature may use trustworthy AI and responsible AI (RAI) interchangeably, but they differ in the underlying intent. Responsible AI addresses the moral, ethical and social consequences of the developed and deployed AI systems.
Read more: Three major concepts that help in understanding Responsible AI.
The first concept of blameworthiness ensures that AI is treated as an agent which can be blamed for a negative consequence. While it may seem unfair, AI algorithms have direct causal connections with outcomes. The developers might be assigned the role of moral agents though.
The second concept is accountability, where an AI system must act responsibly to fulfill an action.
The third concept is liability, where legal responsibility is realized through clear liability assignment in case of a negative outcome. For instance, Uber accepted the liability of death caused by its autonomous vehicle and compensated for it.
All of the above expectations of RAI are achieved through trustworthy AI, secure AI, explainability, privacy compliance, and risk control, among others. Therefore, trustworthy AI is one of the means of achieving responsible AI.
Achieving Trustworthy AI
Approaches to achieve trustworthy AI
It is possible to develop AI solutions that a stakeholder can trust. We present some feasible approaches that can pave the way for trustworthy AI.
It is about the human
Is your deployed model accessible by and representative of different demographics? If not, then data augmentation and assistive features should be incorporated. The model outputs should cover potential user diversity. Even during testing, the model should be exposed to various users and situations during testing. Their feedback should be used to enhance the model further. Additionally, any anomalous output or adverse feedback could prove crucial in uncovering potential issues. Lastly, the user interface should be able to cater to individuals across the capabilities spectrum.
Do not stick to limited metrics
We have already introduced you to the accuracy-fairness tradeoff. A privacy-utility tradeoff is another impacting factor during the model development. Additionally, some notions, such as individual-level fairness against demographic parity, might be contradictory. Therefore, teams should use several metrics to better interpret the tradeoffs. Also, using metrics that measure user feedback and short-term and long-term model performance across different sub-groups can be beneficial.
It also helps to understand the relevance and eventual goal of your model. For example, a fraud detection system should have a high recall. An occasional false alert is better than letting fraud slip through.
Put the data under the microscope before you cook it
A model learns from the training data and its learning is enforced through testing. Therefore, training a model with imbalanced or biased data will cause an unfair model. This consideration is critical even when you have raw data at hand. For raw data, the teams must ensure privacy compliance through anonymization and other appropriate privatization. Moreover, the data should be checked for missing values, sampling errors, imbalance, and incorrect labels.
The acquired dataset should be checked for training-serving mismatch. For instance, training data collected during the summer may not be relevant for a model expected to function over all seasons. The validation data too should be representative of different users and use cases. Feature engineering could prove to be crucial in ensuring trustworthy AI by focusing on significant attributes required by the model.
Lastly, versioning and maintenance of the training testing data can help watch out for data drifts.
Forge your model through testing fires
A trustworthy AI model should be prepared for the unpredictability of the target environment. The use of Continuous Integration and Continuous Delivery (CI/CD), and other DevOps practices can ensure a reliable AI system. While rigorous testing at the unit level is important, integration tests can reveal any discrepancies at the higher system level.
The monitoring of inputs in the target environment can help catch input drift and also strengthen the test plans. Furthermore, test suites that consider the variety of user demography are a good indicator of a team with foresight.
A watched pot seldom boils
A deployed model will face issues due to multiple unforeseen reasons. It is therefore a good practice to monitor your AI system and dedicate resources to answer any raised alerts. The teams should consider the best solutions based on the monitoring reports rather than devising quick fixes. Moreover, approaches like A/B testing and canary deployments can help you check for any issues introduced by model enhancement.
Lastly, monitoring is not complete without explainability reports. While a deployed model may work well, there is always a scope for refinement and the possibility that a specific data subset might cause performance degradation. Explainability reports can help teams regarding such issues.
The Tools to Achieve Trustworthy AI
The tools to realize trustworthy AI can be categorized as follows
Educational tools for trustworthy AI
These tools aim to disseminate awareness and improve the skills of the stakeholders. Some approaches include guidance for trustworthy AI systems design, educational content such as this blog, and awareness-building tools. These tools may also be tailor-made for different stakeholders such as UX designers, data scientists, MLOps engineers, and so on. Moreover, gamification is also a popular and effective method of knowledge dissemination.
The data ethics toolbox founded by the Danish government is a toolkit to guide organizations for data governance incorporation in their products. A research project named Virt-EU is also successfully sharing practical resources to build ethically-sound AI projects. The project encompasses virtues, care, and the capabilities approach.
Procedural tools for trustworthy AI
These tools enable the operational aspect of guidelines which may include governance and management frameworks, risk assessment, product development lifecycle, and process specifications. Procedural tools cater to a wider audience like policymakers.
The IEEE Standards Association (SA) shares recommended practices to assess the impact of AI on human well-being. Another offering by IEEE SA is a playbook for the financial sector to guide curation of the trusted data and best practices. Another contribution of IBM research toward trust in AI by increasing transparency and enabling governance can be found in the AI Factsheets 360. It is a repository of information tailored for different stakeholders and models to foster AI governance.
Technical tools for trustworthy AI
These tools or commonly called toolkits address technical requirements such as transparency provisions, explainability, bias detection, performance monitoring, and security measures. Some of the popular technical toolkits include LinkedIn Fairness Toolkit (LiFT), Microsoft Fairlearn, Google Cloud Explainable AI Service, and Microsoft InterpretML among others. While these are the offerings of the big tech, the open-source community has also developed frameworks to provide reliable, transparent, and accountable AI systems. These include the EUCA and the STAR frameworks. You may read more about them in this informative comparison discussion.
We at Censius are also working towards bringing trustworthy AI to organizations of different scales and visions.
We appreciate the varying needs of stakeholders and offer an interactive, easy to setup environment to get you started in the right direction.
The Censius AI Observability platform not only answers your model monitoring and data governance needs but also brings transparency through explainability reports. Early bias detection and cohort-specific analysis will make your model robust against unforeseen issues.
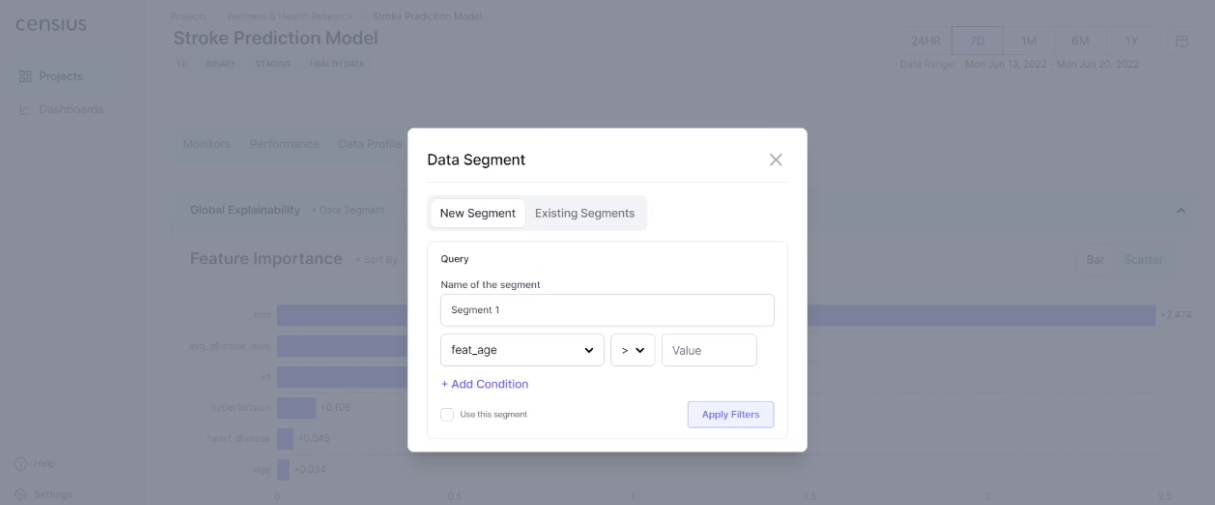
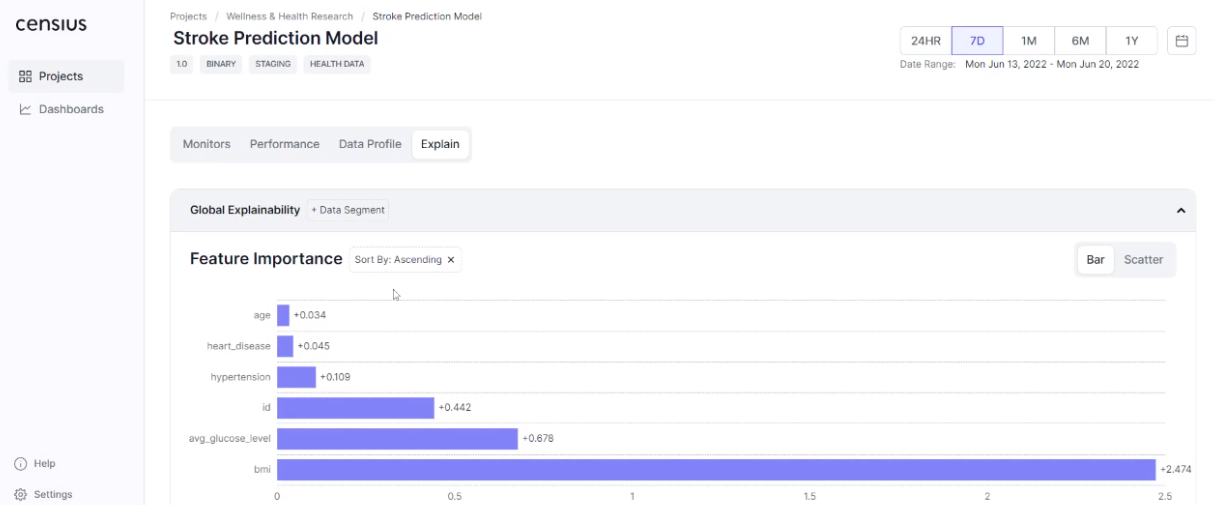
If we have got you curious, then let us show how Censius can elevate the trustworthiness of your AI project by starting this demo.
Wrapping up
Trustworthy AI is a step above conventional computing due to various factors like data governance, human rights, and the fact that AI-powered decisions could be critical. It is a push toward AI systems that are transparent, accountable, safe, sustainable, and fair. While the approaches could range from educational guidance to operational specifications, the technical methods prove to be the most relevant. The ability to incorporate safe practices during development and deployment can ensure a reliable AI product.
- Trustworthy AI
- Establishing the rules for building trustworthy AI
- Responsible AI and Its Stakeholders
- Responsible AI practices
- Tools for trustworthy AI

Explore how Censius helps you monitor, analyze and explain your ML models
Explore Platform