Let’s start with a story.
Scenario 1
Merry gets her physical checkup done every year. This year also, her checkup report was normal. She continued her routine happily with work, exercise, and stress management.
Scenario 2
Harry was not feeling well for a week. He usually avoids hospitals, but this time he was helpless. He went to a physician and performed all prescribed tests. The test report indicated high cholesterol. What next? His future seemed full of stress, with restrictions on his favorite foods.
Would you like to be in Merry’s situation or Harry’s? You’d probably want to be in Merry’s position if you love food like me.
Can you imagine the same situation for ML models? Let me explain.
You work hard to research, build, and deploy your ML models. Models perform well for some period, but after some time, these start degrading. ML model prediction accuracy gets hampered, and your assets become liabilities.
Imagine that you have an ML model monitoring system for monitoring ML models in production. Now, you can take proactive actions and strategize to ensure the required quality of ML predictions.
What do the Numbers Say?
S & P Global Market Intelligence surveyed different tech leaders and laggards to get more insights on model management.
ICYDK: Tech laggards are industry stakeholders who take ‘wait and see’ or adopt a late approach.
Here are some interesting findings from their research:
- 32% of respondents replied they are currently using a monitoring or incident response tool for AI/ML, with a further 12% in the research or proof of concept stage.
- 39% of tech leaders are using AI/ML monitoring tools, compared to just 11% of tech laggards.
- 19% of tech laggards admitted that they are considering ML monitoring tools but have no current plan, whereas 16% of tech leaders are considering ML monitoring solutions seriously for the future.
ML has gone mainstream with increased dependency on ML frameworks and monitoring tools. These tools help avoid prediction errors, visualize datasets, monitor models, and share feedback. Analytics Insight highlights that the market for these tools is expected to grow by US$4 billion.
How are Modern Industries Practicing ML Monitoring?
Monitoring ML models is a high priority for modern ML-driven organizations. Investing in ML monitoring solutions is beneficial to drive expected returns with your AI investments.
ML model performance monitoring helps reduce failure incidents and offers timely remediations. It involves tracking issues such as training-serving skew, concept and data drift, upstream data issues, data quality, model activity, and performance metrics. We researched a bit to find how different industries are practicing ML monitoring. We found:
- Netflix, Intel, Intuit, Doordash, Uber, Booking.com, Etsy, and Pinterest - all these companies have considered ML monitoring to be crucial in their MLOps implementation. These companies have implemented model monitoring solutions to serve customized monitoring needs and capabilities. These companies developed monitoring systems in-house using open-source tools.
- Doordash, Booking.com, and Uber's ML monitoring systems track the distributions of model inputs and outputs over time. Such capability helps detect data issues that occur due to changing distributions. These tools also enable alerts on feature distribution shifts.
- A monitoring system designed by Netflix empowers data scientists to schedule their customizable notebooks to monitor deployed models.
- Intuit has a service that helps data science teams define monitoring pipelines through config files.
Monitoring model quality is context-specific and mostly served by customizable monitoring toolkits or platforms.
On the other hand, monitoring operational metrics has become easy as many monitoring platforms offer these features inbuilt.
Exploring the Build vs. Buy Scenario for ML Monitoring
Machine learning is snowballing and transforming industries incredibly. But the main concern is how to maximize your ML investment returns? Is it better to build the tooling and infrastructure required to monitor ML projects? Or is it wise to buy readymade solutions to fuel your ML initiatives? ML decision stakeholders have to choose the right approach. Let us explore both arguments.
Building an ML monitoring solution
Building an ML monitoring platform in-house sounds interesting. But decision-makers must consider different factors to operationalize their custom-built in-house ML monitoring solution. These preferably include
- team resources
- scope
- cost
- timeline
- development requirements
- infrastructure
Also, the efforts required to build, deploy and maintain such a platform should not be underestimated for a fair comparison with another approach - buying an ML monitoring tool. The do-it-yourself approach for building an ML monitoring tool prioritizes answering these questions:
- Do you have a limited timeframe to set up your ML monitoring infrastructure? Can you afford ML monitoring solution deployment delays to offset the costs of buying a readymade solution? Will it have any further consequences, such as your competitors getting a plug-and-play ML monitoring solution and getting ahead of you?
- Do you have sound and consistent team support to build, deploy and manage ML monitoring solutions in-house?
- Are you sure that developing an ML monitoring solution optimizes your organization’s budget and time?
- Is your ML monitoring platform capable of serving all your model monitoring needs? Is it supporting future needs of AI initiatives, such as AI explainability and observability?
- What resources are required to build, launch and manage the monitoring system?
- Which crucial aspects are considered regarding the data, models, and infrastructure security while building an in-house ML monitoring solution?
Building an ML monitoring tool in-house becomes challenging with the current economic uncertainty, scarcity of the right talent, and the state of hiring. Here are some other key considerations applied to in-house ML monitoring solutions.
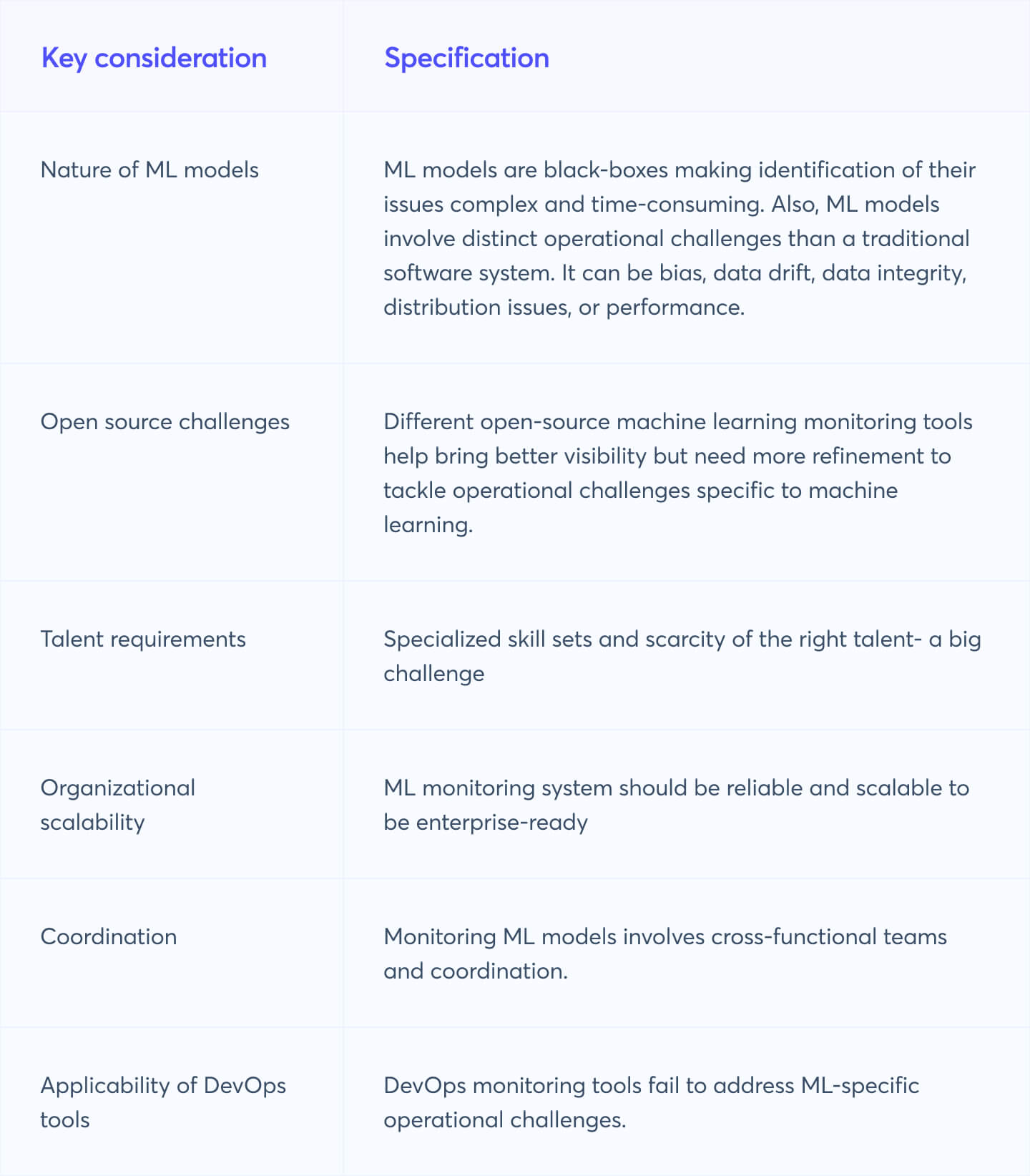
Buying an ML monitoring solution
Many enterprises prefer buying ready-to-use and proven ML monitoring platforms instead of in-house ones. Such readymade monitoring solutions drive multi-fold benefits – faster monitoring system deployment, teams can focus on core tasks, no planning delays, low maintenance costs, and more. A buying decision for an ML monitoring system requires considering the following questions:
- Does the ready-to-use solution integrate with your current tech stack and workflows?
- Is it scalable to serve enterprise-grade ML monitoring needs?
- Is it scalable to support AI explainability and other observability features to build a sustainable and strong monitoring system?
- Will it add positive business value to your organization?
- Does it support better collaboration, visualization, and other relevant capabilities?
- Is it serving monitor alerts for all your requirements? Is it possible to customize the solution for segmented analysis or similar alerts?
- Will it help save costs and time?
- How efficient is the process of configuring the ML monitoring system? Is it as easy as –plug and play? Or does it involve complex settings and dependencies on vendors?
- Is the solution competent and aligned with your enterprise security and compliance requirements?
- Is this ready-to-use monitoring platform within your budget?
- Is this monitoring solution future-proof and helps avoid lock-in?
Build Vs. Buy for ML Monitoring Solutions
While exploring buy vs. build for ML monitoring solutions, we must understand both options' advantages and challenges.
.jpeg)
Selecting the Right Approach
After discussing both buy and build options for ML monitoring solutions, the next question is 'Which is the right path for you?'
Building an ML monitoring platform in-house, though attractive, is restricted by several challenges. Slow adoption of monitoring systems can prove expensive in terms of competitive advantage. Specialized talent acquisition and maintenance costs hit your purpose of maximizing ML investment returns.
On the other hand, buying an ML monitoring solution like Censius helps you save time, resources, and maintenance overheads. The Censius AI Observability Platform monitors the entire ML pipeline, explains predictions, and enables you to take proactive actions. It allows setting and customizing ML solutions for data quality, drift detection, model activity, and ML model performance monitoring. More importantly, it brings accountability and explainability to your models in addition to mere monitoring.
I hope this article helped you understand buy vs. build scenarios for your ML monitoring solutions.
The Censius team would be happy to discuss further if you have any queries. Just drop an email at hello@censius.ai or sign up for a demo.

Explore how Censius helps you monitor, analyze and explain your ML models
Explore Platform