Algorithms are made to mimic human learning capabilities and decision-making. While there is still a long road to achieving sentient beings of sci-fi movies, learning programs are already simulating the biases typical of the human race.
According to Cathy O’Neil, author of Weapons of Math Destruction, algorithms can enforce negative social norms that include spreading bias. While the said bias could be explicit due to malicious intent of the developers or users, mostly, it is the oversight that may cause biased logic.
Bias is a broad word thrown around by fighting siblings and referee-like parents, but in Machine Learning (ML), it causes more profound problems. Imagine that you wanted to train a neural network to classify between pizza and burger. Of course, you need an image dataset for that, and your friends and family need to oblige by sending pictures of what they usually order. Now certain brands are preferred in your town, and all these pictures were clicked while the food was in the box. The AI model might consider the box colors and icons specific to those brands as important features for classification. In this case, the model acquired bias from the humans who provided the training-testing datasets.
Biased ML around Us
An ML model biased towards specific pizza and burger makers is not a critical problem unless you are planning to sell the same snacks. Now imagine a learning algorithm that predicts default risks of loan applications or predicts if the handheld object in the pictures is a gun or not. Let us also assume that the logic is favorable to a skin color or gender above others. Now, dear reader, you have a bigger problem at hand.
Take these instances in the recent past that throw light on ever-increasing bias in learning algorithms:
- In 2016, an AI-based Twitter chatbot built by Microsoft was poisoned with racial and chauvinist language. Its learning logic meant to create interactive tweets was abused by some people.
- Recently, Correctional Offender Management Profiling for Alternative Sanctions (COMPAS) emerged as the most alarming instance of racial bias in AI systems. The algorithm assigned risk scores for a defendant's likelihood of committing another offense. The scores on the high, medium, and low risks scale were computed using 16 attributes such as substance abuse and residence. The algorithm was found to be partial towards the white defendants and assigned low scores to most of them.
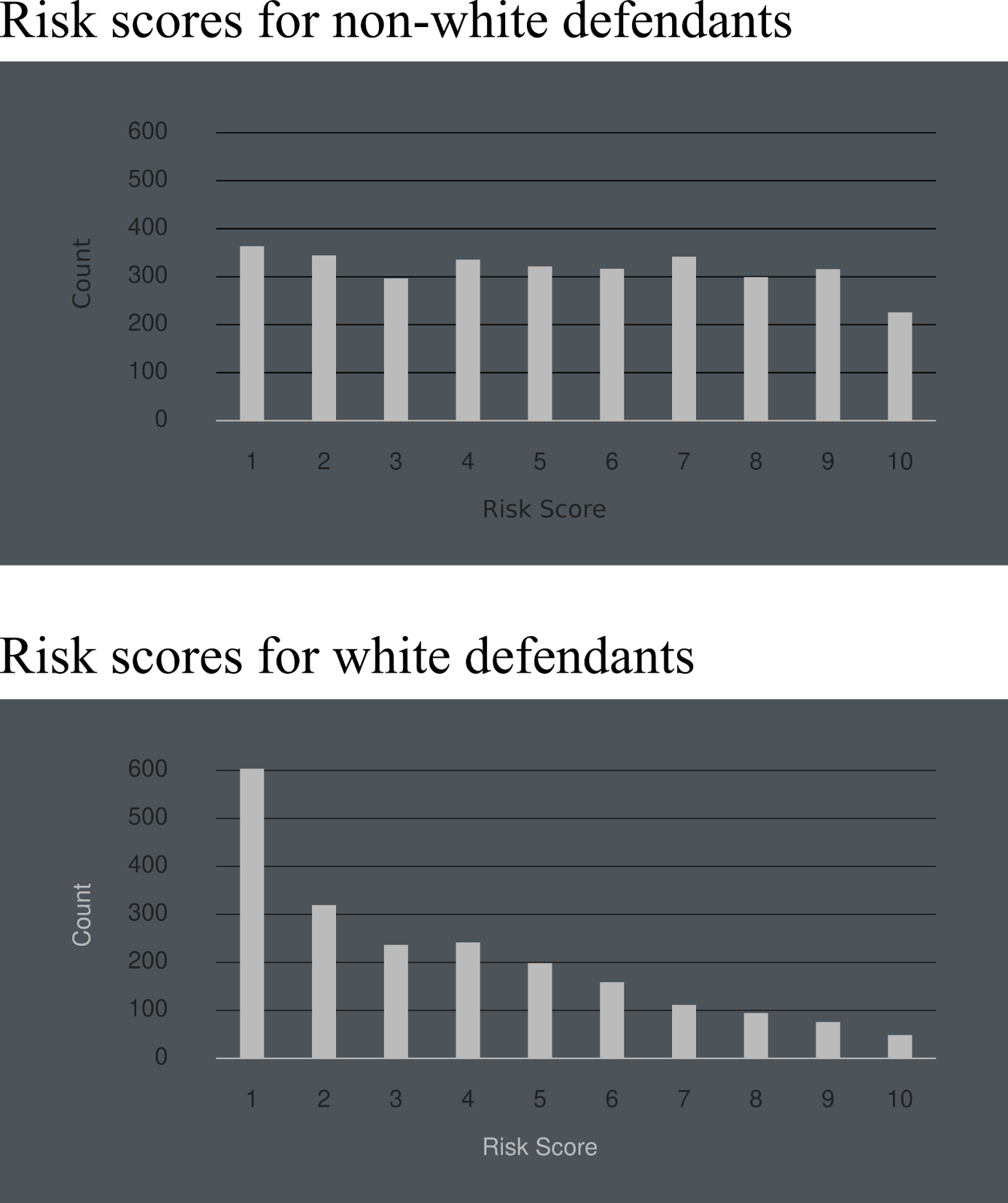
- Another learning algorithm used in the judicial domain called PredPol highlighted how AI systems walk on thin ice. The algorithm predicted the localities that had a high chance of crime occurrence and was adopted by police departments in many US states like California, Maryland, Florida, etc. Its feedback-based logic caused bias since it used the number of police reports filed in the area while ignoring correlated factors like the concentration of law enforcement. The predictions by the algorithm were found to target neighborhoods with larger densities of racial minorities.
- In 2015, the algorithm used for hiring decisions at Amazon was found to be biased against women since its training dataset collected over the past decade had majorly comprised of males. The imbalanced training dataset caused an embarrassing instance of gender bias.
- The critical domain of healthcare is also not free from biased ML. In 2019, racial bias was uncovered in the algorithm that predicted which patients should be given extra medical care in US hospitals.
What Laws have to say about Algorithmic Bias
With such major implications, it is required to rein in the potential for bias among ML systems. More so, to set regulations to guide the development of impartial algorithms.
The Federal Trade Commission (FTC) has been revamping numerous laws to include AI. For instance, the Fair Credit Reporting Act (FCRA) was passed in 1970 and regulated the collection and sharing of information by businesses. The act was updated in 2021 to ensure that agencies using AI must not misuse data and algorithms while making consumer-centric decisions. In the words of Andrew Smith, the director of the FTC's Bureau of Consumer Protection, "the use of AI tools should be transparent, explainable, fair and empirically sound."
Another regulation under FTC guidance that has been updated for AI practice is Equal Credit Opportunity Act (ECOA). The act prohibits credit discrimination based on race, gender, religion, and age, among many factors influencing the decisions taken by AI-based algorithms.
In May 2021, Algorithmic Justice and Online Platform Transparency Act was introduced to curb algorithms hosted on websites to snoop on user activity. Though, the act does not address housing, employment, or credit rating calculations.
In a more recent event, the Biden administration will be advised by the National Artificial Intelligence Advisory Committee (NAIAC), which was announced in April 2022. In the inaugural meeting, the committee debated issues related to U.S.-specific AI practices, workforce laws, and required regulations over AI systems.
The committee is a firm step toward AI regulations since FTC can only respond to complaints or reported violations. Its laws have been updated to include AI regulation but are not solely focused on them.
Where do You Figure in Curbing Bias
Some of the ways you can lend a hand to the cause of more responsible AI:
- Foster AI regulations awareness by joining discussion forums, hosting and attending workshops, and encouraging dialogues between legal and development teams.
- The inherent imbalance and bias in the training-testing datasets greatly influence the learning algorithms
- Set data integrity checks and compliance practices among your team.
- Use synthetic datasets to address the class imbalance that may contribute to bias.
- Transfer learning and decoupled classifiers on different groups hold the potential to reduce bias for facial recognition algorithms.
- The model evaluation methods should be inclusive of different groups.
- AI systems are still dependent on human judgment. Sensitization and awareness among the involved teams can help curb bias issues.
- Invest more in practices that can lower the bias potential in your projects. Some examples:
- Extensive data collection across groups and checks on acquired datasets
- Explainability reports that can be easily generated using services of the Censius AI observability platform
- Tests to check the fairness of algorithms
Wrapping up
ML algorithms mirror the beliefs and practices of people who develop them. A plain oversight, imbalanced training sets, failure to test models across different groups, and vulnerable logic can cause unintentional bias. Additionally, the training logic of the systems can be abused to hurt focus groups. Laws are being tabled to ensure that consumers are not denied services based on AI-driven decisions. But it is just the beginning of a long journey. You can also play a crucial role in ensuring that impartial systems become a part of the customer experience. Incorporating the shared practices would help your teams deliver more inclusive projects.
PS: Ready to detect and prevent bias in your machine learning models? Try Censius AI Observability tool now and ensure your models are fair, transparent and accountable.
Sign up for a free trial today.

Explore how Censius helps you monitor, analyze and explain your ML models
Explore Platform