Artificial intelligence is arguably one of the most revolutionary technologies being developed today. From data-intensive industrial automation to medical diagnosis, artificial intelligence is starting to influence every aspect of our life.
But there's one major stumbling block to the widespread adoption of AI - people don't trust it.
We're more likely teaching a human how to use a new machine than trusting the machine to do its job without supervision or interference. We need to make our models more trustworthy and reliable.
This post will discuss what AI model explainability is, why it's essential, and how to scale it.
What is Explainable AI?
Explainable AI is a research field on ML interpretability techniques whose aims are to understand machine learning model prediction and explain them in human and understandable terms.
- It is a vital part of broader human-centric responsible AI practices.
- It focuses on model explanations and the interface for translating these explanations into human, understandable terms for different stakeholders.
- In simple terms, it's as if you're asking, "Why did the AI do that?"
Explainable AI, or XAI for short, is not a new term; it dates back to the early days of artificial intelligence research and development. Using XAI to gain a functional knowledge of ML model behavior can provide valuable insights that can help improve the application.
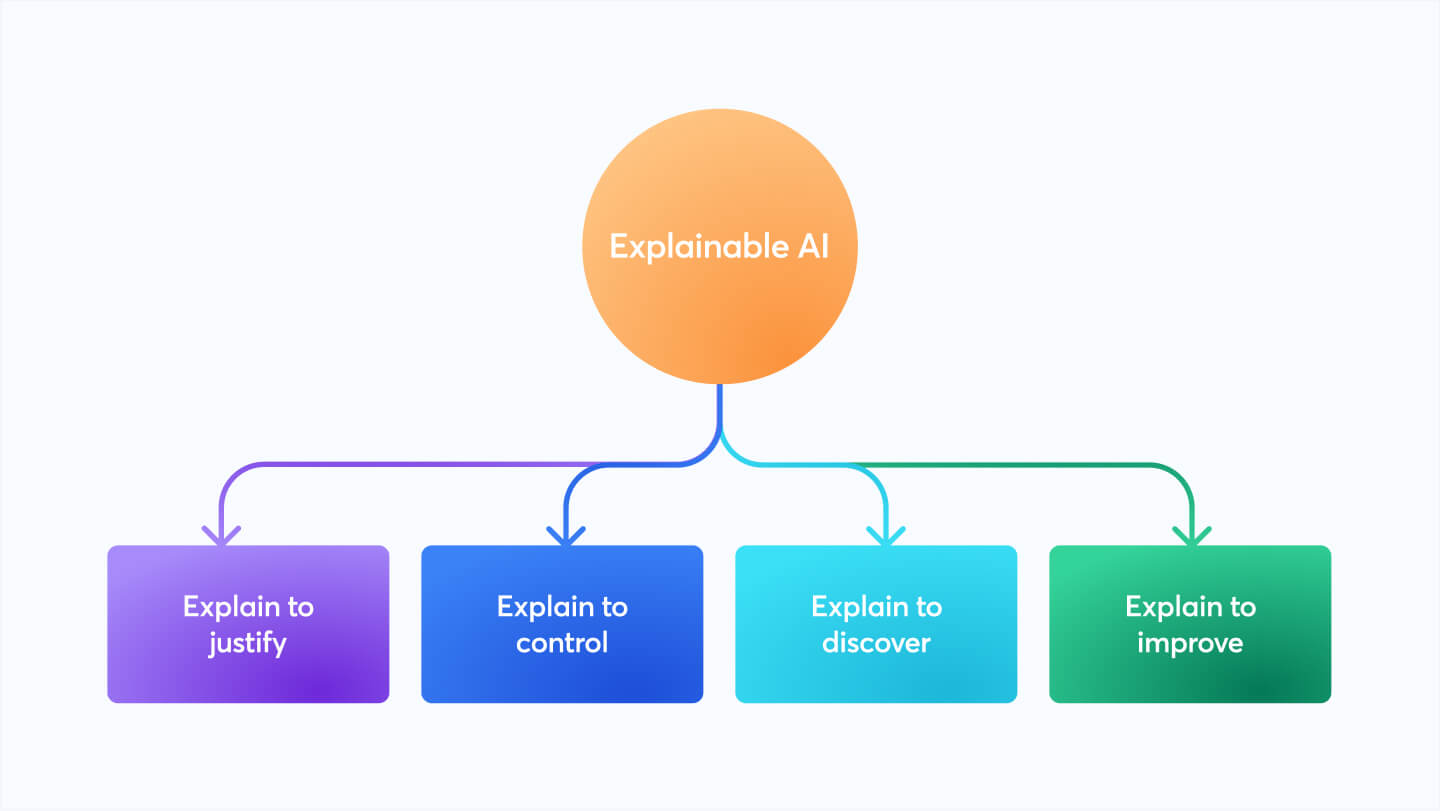
Why is it Important to Understand a Model's Behavior?
- Unexpected behavior in the form model must be debugged.
- Refine modeling and data collection processes
- Explain predictions to help in decision-making.
- Verify if the model's behavior is acceptable.
- Stakeholders should be informed about the model's predictions.
Model understanding for different stakeholders
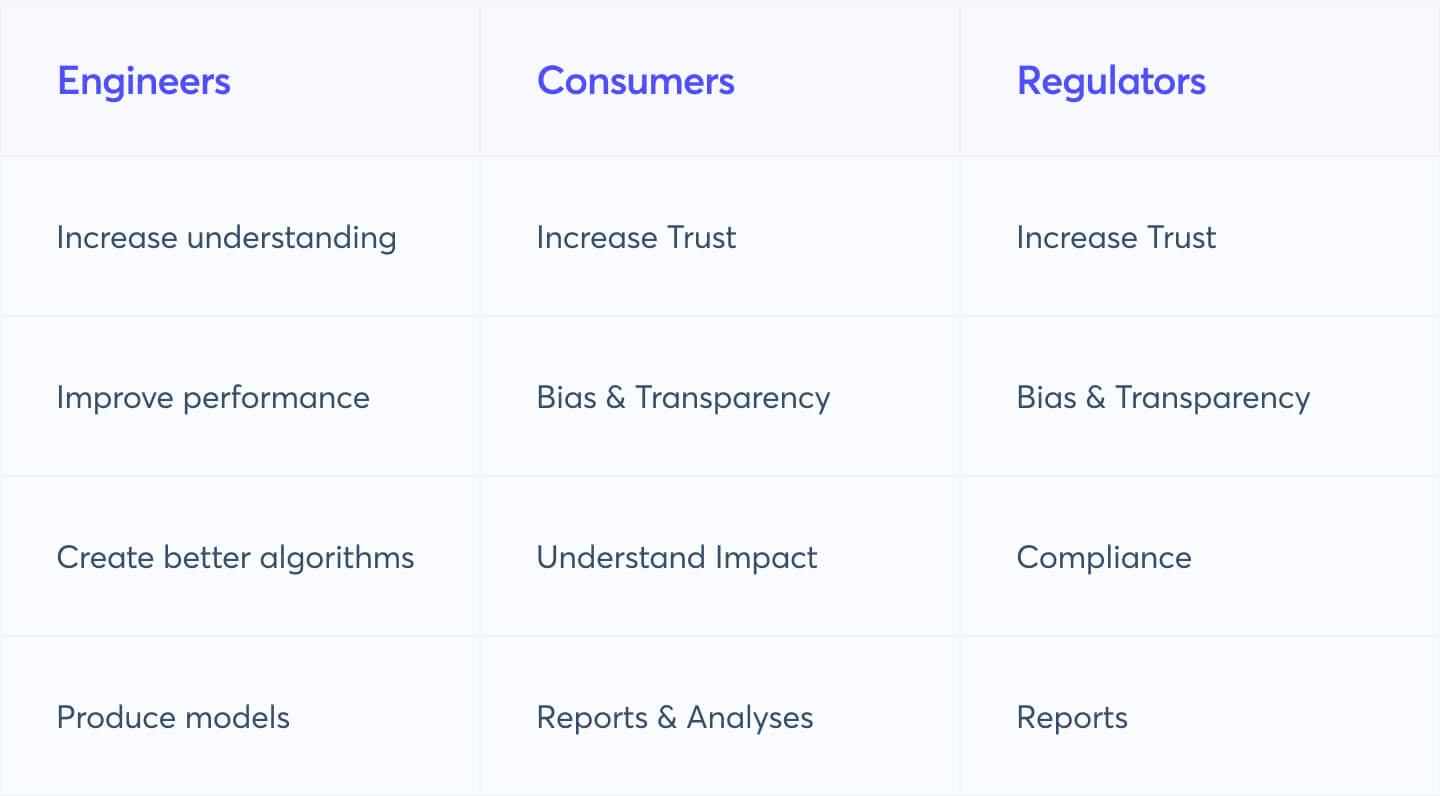
Why do we Need Explainable AI?
Explainability is frequently overlooked in machine learning.
A machine learning engineer can create a model without ever thinking about how explainable it is. In simple terms, it's similar to a car's seat belt; it doesn't affect the vehicle's performance, but it protects you in an accident. Let's take a look at a couple of benefits of explainable AI.
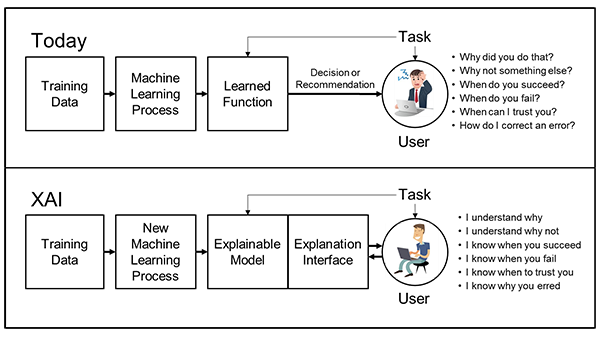
Benefits of explainable AI
Bring Down the Cost of Mistakes
In decision-sensitive industries such as healthcare and finance, errors in precision have a significant impact. Oversight of the outcomes decreases the effect of incorrect outcomes while also identifying the main problem, allowing the underlying model to be improved. You can bring the cost of errors down using XAI.
Implement AI with confidence and trust
With XAI, you can boost production AI's trustworthiness, bring your AI models into production as soon as possible while ensuring they are comprehensible and understandable. You can simplify the model evaluation process while improving transparency and traceability.
Explainable AI examines why a decision was taken for AI models to be more interpretable for human users, allowing them to understand why the system reached a particular conclusion or took a particular action. XAI aids in the transparency of AI, possibly allowing the black box to be opened and the complete decision-making process to be shown in a form that humans can understand.
Enhance speed
Explainable AI helps you monitor and manage models in a systematic way to improve business outcomes. You can evaluate and enhance the model's performance on a regular basis.
Reduce the risk of model governance
XAI helps you keep your AI models simple and easy to comprehend. With XAI, all regulatory, compliance, risk, and other requirements are met. It reduces the time and money spent on manual inspections, and the chance of unintentional prejudice.
Performance of the model
Understanding possible flaws is one of the keys to maximizing performance. It is simpler to enhance models if we have a better knowledge of what they do and why they occasionally fail.
An Explainable AI tool will help you spot model faults and data biases, and it helps users trust the model’s decision making. It can aid in the verification of predictions, the improvement of models, and the discovery of fresh information about the topic at hand. Understanding what the model is doing and why it makes its predictions makes detecting biases in the model or dataset simpler.
Scaling AI with XAI
Let's have a look at some interesting strategies to implement, promote and scale XAI in an organization.
Prediction accuracy tools
Accuracy is a critical component of AI's performance in day-to-day operations. Simulations and comparisons of XAI output to the findings in the training data set can be used to evaluate prediction accuracy.

There are many Techniques for explainability, but Local Interpretable Model-Agnostic Explanations (LIME) is most used in this case. LIME measures the consequences of multiple multi-feature disturbances around a certain prediction. It illustrates how the ML algorithm predicts classifiers. LIME uses a series of experiments to try to reproduce a model's output. SP-LIME, a method for picking representative and non-redundant predictions, was also created by the developers, giving users a global perspective of the model.
Traceability approach
Another important strategy for achieving XAI is traceability. This may be accomplished by restricting the kind of decisions that can be made by limiting the scope of ML rules and features.
DeepLIFT (Deep Learning Important FeaTures) is an example of a traceability XAI approach, It compares each neuron's activity to 'reference activation via backpropagation, then records and allocates a contribution score based on neuron differences.
Decision understanding among developers
Data scientists and machine learning engineers commonly make the mistake of thinking about explanations from the perspective of a software developer rather than from the standpoint of the end user.
This is when the human aspect comes into play. Many people are skeptical about AI, yet in order to work effectively with it, they must learn to trust it. This is performed by training the AI team so that they are aware of how and why the AI makes the decisions it makes.
Using KPIs for AI risks
When analyzing machine learning models, businesses should evaluate the particular reasons for using explainable AI approaches. Teams should define a set of KPIs for AI risks, which should include comprehensiveness, data protection, bias, transparency, explainability, and compliance.
A benchmark should be established by organizations. This will allow businesses to compare their AI operations to industry standards as well as build their own best practices.
Recommended Reading : List of Techniques for explainability
Explainable AI Use Cases
The benefits of XAI may be found across a wide range of sectors and job activities. Let's have a look at how XAI would benefit the healthcare and finance sector.
Healthcare
Problem : In the healthcare business, ML and AI are already being used and applied. AI is used in a wide range of medical fields. Doctors, on the other hand, are unable to explain why models make particular decisions or projections. Healthcare is a field that demands a high level of accuracy. As a result, AI technology can only be employed in certain ways and in certain places.
Solution: Doctors can utilize XAI to figure out why a patient is at such a high risk of being admitted to the hospital and what treatment is best. As a result, doctors may make judgments based on more reliable data. It also improves transparency and traceability in clinical decisions. With XAI you can also speed up the pharmaceutical approval process.
Finance
Problem: Financial institutions and platforms are using AI in many ways. They aim to give financial stability, knowledge, and management to its consumers. They still have issues with regulatory compliance, customer trust, and a variety of other issues. Many times, the system misidentifies the customer and declines a particular service or wrongly reports something. As a result, the consumer is disappointed and loses trust in the business leading to negative impact on business reputation and loss of customers
Solution: XAI can be used by financial services to provide clients and service providers with fair, unbiased, and transparent outcomes. It helps organizations to comply with regulatory standards while remaining ethical and fair in their operations. XAI will also improve the customer experience and reduce the time it takes to resolve complaints by using a simple automated processes
Recommend Reading : Explainable AI Driving business value through greater understanding
Why is AI Explainability the Next Big Thing?
AI is progressing, deployment is expanding, and the attention of regulators, consumers, and other stakeholders is growing in lockstep.
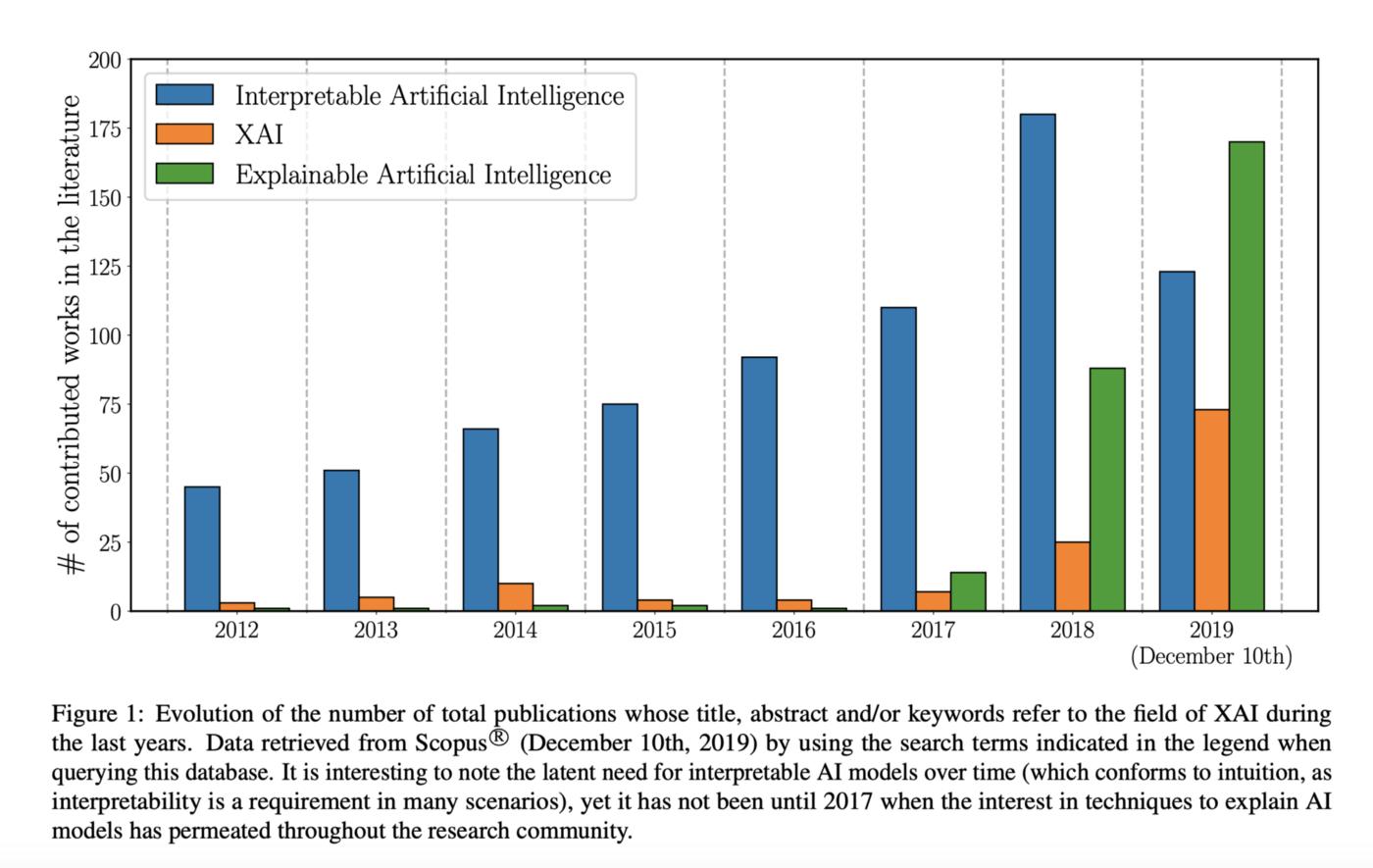
- Developers are primarily concerned with meeting well-defined functional requirements, whereas Business Managers are concerned with measuring business indicators and ensuring regulatory compliance. Explainable AI can bridge the gap between non-technical executives and developers, allowing top-level strategy to be effectively communicated.
- The need to be interpretable is becoming more important. In industries like financial services, the use of sophisticated AI is already well-established. Other sectors, like healthcare and transportation, are catching up quickly. Executives in companies should be aware of the risks of Black-Box AI. As a result, executives must ensure that a system operates within specified parameters. XAI will help build confidence in the models.
- XAI will enhance the dataset, which will increase the model's accuracy. It'll inform us which parts of the input are responsible for the model's decision. As a result, we can determine whether our dataset has any bias or discrepancy. If that's the case, we may create dataset preparation rules, which will increase our model's accuracy.
Although it is still in its early stages of development, the research community and industry have grown at an exponential rate since then. The healthcare, defense, transportation, and banking sectors all require significant improvements. There is a steady stream of innovation and regulatory laws that will aid in the implementation of XAI and its applications.
Explainability AI's limitations
Explainability is influenced by the sort of learning method used to create a model. However, the sort of explanation needed and the type of input data utilized in the model can be critical. Because the XAI is a relatively new field of study and development, the community lacks a uniform metric, benchmark, or transparency.
Problem domain
The majority of explanations consist of a list or visual depiction of the most important factors that affect a decision and its strategic significance. Certain problems are difficult to comprehend just by quantifying a few factors.
Data preprocessing
To increase accuracy, many ML systems use extensive data pre-processing. When organizations use word vector models on textual data, the data's original human meanings may be obscured, making explanations less helpful.
Correlated input features
When strongly correlated training data is available, correlations are usually lost or simply overpowered by other more predictive correlates throughout the feature selection process. As a result, uncertainty about the latent component behind the correlates is hidden from the explanation, perhaps leading to an inaccurate conclusion for the stakeholders.
Using Censius AI Observability Platform to Deal with Explainability
With Censius teams can design higher performing and responsible models by explaining model decisions, which will boost confidence among all stakeholders. It will help you can:
- Enhance the performance of certain cohorts.
- Detect unwanted bias and fix models.
- Understand the “why” behind model decisions.
- Ensure that models stay compliant.
Consider a loan disbursement model for granting loans to businesses. Let the data variables for this model be the company's industry, yearly revenue, and total annual items sold to keep things simple.
Now, the model may give more weight to the amount of items sold. This, on the other hand, may not be a wise decision. The model may provide more credibility to a company selling 10,000 goods for $10 than a company offering 5,000 products for $100.
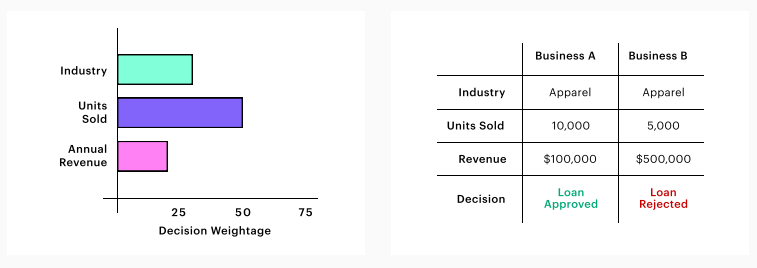
The Censius AI Observability Platform will assist you in identifying such blind spots in your model's decision-making so that you may correct them by effectively training your models. You may also figure out why your loan application was turned down and what went wrong with your model.
In the event that the model makes a mistake, it also allows for the discovery of a faulty relationship between the model's input and output, which may then be addressed. It will help businesses keep their model decision making processes under control by assisting them in analyzing model outputs that exceed a specified threshold or baseline.
Schedule a demo to understand how the Censius AI Observability Platform can help your models set up for success.
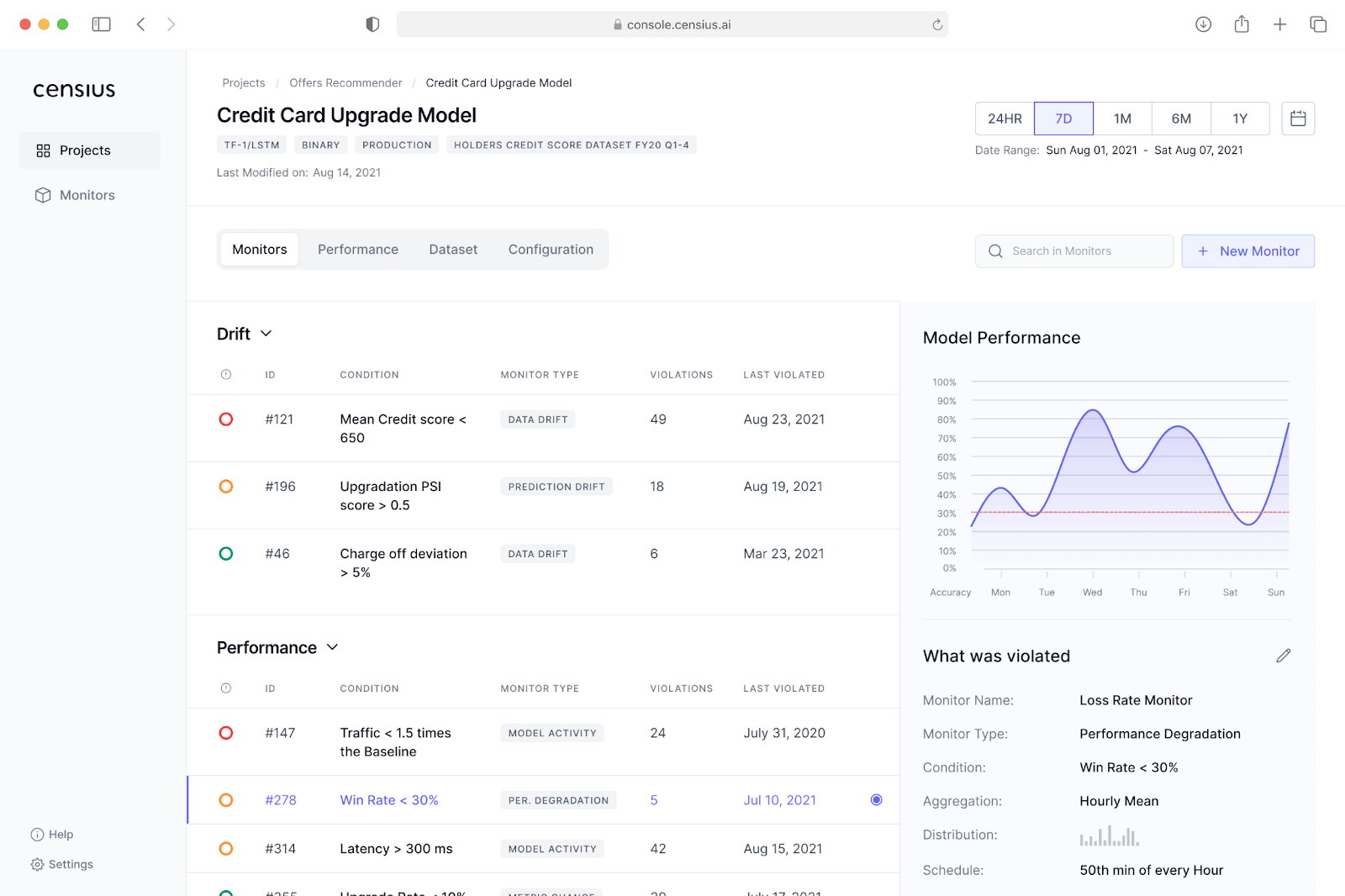
Conclusion
Having an explainability solution like Censius in place can assist businesses in maintaining their confidence as they pursue innovation. Explainability may be a critical first step in opening the AI black box and making model decision-making transparent to all stakeholders, especially when governments place strict laws around technology.
Explore Censius AI Explainability Features

Explore how Censius helps you monitor, analyze and explain your ML models
Explore Platform