AI is one of the most forward-looking technologies in today’s time, and it promises to have a profound impact on our way of life. However, before it can truly take off and make a difference, there are practical concerns that need to be addressed.
There are different concerns related to AI tools and technologies. Conversely, AI itself has been criticized for biased engineers’ decisions due to inherent bias from automation processes in algorithms. This article aims to share key insights into what practical steps can be taken towards achieving sustainable success in this emerging space by addressing some critical issues within these concerns. Before we explore several strategies for achieving sustainable AI, let's talk about the factors businesses should consider while developing an AI roadmap.
Leaders must focus on four essential elements to create effective AI roadmaps.
- Create a compelling and convincing narrative - Demonstrate what artificial intelligence is and why it is important for the organization's future. You must explain to the stakeholders how AI will contribute to the long-term success of your company.
- Spend time and effort addressing the challenges- There are many challenges to adopting agile ways of working, concerns within the workforce about becoming outdated, etc. The leadership group must present a vision that unites everyone and demonstrates how each individual fits into the new AI-driven culture.
- Financial Planning- Planning should be done for everything, including technology, training, and implementation. Most profitable businesses devote more than half of their analytics spending on adoption-promoting initiatives, including workflow reform, communication, and training.
- Choose and evaluate the appropriate use cases- Even while the emphasis shouldn't be solely on securing rapid victories, pursuing projects that might not be implemented or yield no immediate benefits might seriously harm present and future AI chances.
Let's look at a few strategies you may use to ensure the long-term success of your company.
First, the problem, then the solution
Organizations often make many mistakes while planning business goals. Many ML companies mistake aligning their efforts with a technical aim rather than a strategic business goal when it comes to AI. Consider the large-scale objectives your company is pursuing, such as increasing revenue or lowering expenses, and look at where and how AI might help you reach those goals. The efficiency and reliability of AI will make the most significant impact in achieving your company's objectives.
Many AI professionals, particularly executives, tend to search for current use cases used by businesses like their own or those carried out by vendors, then try to implement them within the company. But this strategy not only misses out on greater AI application options but also disregards the complicated requirements and business activities and procedures. The bottom-up method, in which practitioners strive to comprehend the company's focus areas, issues, and procedures before creating personalized AI solutions, is a better strategy that results in long-term success. AI implementation strategy will help you:
- Identify trends and opportunities in the future
- Set business priorities.
- Understand budgeting.
Boosting Team Skills and Diversity
Global and regional functional leaders must understand how to choose the relevant themes in their functions to use AI, allocate yearly budgets for AI initiatives, create new KPIs to assess AI performance, and more. These functional leaders must also be aware of the challenges, such as cost and effort. Things to keep in mind:
Consider data engineers and scientists as partners in business
The need for meaningful business collaboration with Data Scientists is sometimes undervalued, despite the fact that we are accustomed to roles like Business executives or Finance executives in the company. To make your AI goal sustainable, consider your data scientist and engineer as your business partner. As a result, your business will become more data-driven. When combining business operations with the knowledge and expertise of data scientists, you create new opportunities.
Upskilling Team
A skilled and diverse team can make business more efficient. Every executive or artificial intelligence team manager must be trained to integrate the company's strategic goals with the AI vision, determine the AI plan and direction for the company, foster the correct culture, and assess ethical, legal, and other non-technical issues such as workforce effect.
Maintaining a diversified workforce
Diversity must be a part of the design and approach. Focus on selection techniques, for instance, by educating your recruiters in selection processes and objective interviews. Establish a baseline using measures for the present diversity statistics and track the development. You can ask operations teams to create activities and programs, and to hire students or unique talents for big data and data science.
Having the appropriate individuals in place will aid in executing your AI strategy and guarantee that you are utilizing AI in the appropriate areas to achieve your objectives.
Responsible AI
Responsible AI (RAI) creates best practices for developing, deploying, and scaling AI for good reasons and to positively impact individuals and society. It increases people's faith and confidence in artificial intelligence.

With the widespread use of AI systems across many industries, several problems with AI ethics, trust, legality, and data governance have emerged. For example, black individuals are being classified as gorillas by Google's face recognition software.
The inappropriate or sudden deployment of such data-driven initiatives might be detrimental to human rights. Data-driven tactics ought to be responsible in some way. The adoption of ethical concepts together with careful preparation is necessary to create responsible AI. Data-driven choices must be assessed on various fronts, including commercial risks, data privacy, health and safety concerns, and equality.
Taking a responsible approach will enable businesses to
- develop AI systems that are effective and compliant with laws;
- make sure that all societal, legal, and ethical ramifications of AI are taken into account during the development process;
- monitor and reduce bias in AI models;
- create AI trust;
- avoid or reduce detrimental impacts of AI; and
- eliminate any doubt as to "whose fault it is" if an AI-related issue arises.
Now, all-purpose and adaptable Responsible AI is also becoming more popular since its architecture can support various AI applications, such as algorithms for predicting credit risk or recommending videos. Using automated tools for fairness, monitoring, and explainability. AI observability solutions such as Censius AI Observability Platform can help here.
Learn more about Responsible AI
Trustworthy and Secure AI
A sound AI strategy will not be enough to avoid all risks or make AI ethical. AI requires collaboration between teams from several disciplines. To do their job, machine learning engineers and data scientists need complete freedom without jeopardizing security and privacy.
Organizations should have an updated data policy that allows AI professionals to access protected data as necessary. A responsible AI framework requires updating data access regulations and, in certain situations, broadening access by role.
Additional measures to safeguard privacy in AI include:
Apply effective data hygiene: Only the data types required to build the AI should be gathered, and even those should be kept safe and preserved for only as long as required to achieve the goal.
Control: Users should be aware of how their data is being used—if AI is being used to draw judgments about them, and whether their data is being used to build AI.
Additionally, they should have the option to agree to their data usage.
Eliminating algorithmic bias: When "training" AI, ensure that the data sets are extensive and diverse. For comparitively minority populations of the technological workforce, such as women, and the elderly, algorithmic prejudice presents issues most frequently.
Build or Buy
When AI teams embrace a use case, one of the most critical decisions they must make is whether to build the solution or buy the solution. This choice has an impact on the solution's long-term success. Furthermore, purchasing an AI solution without considering the long-term implications may impede adoption and usage, resulting in unhappiness and distrust among company stakeholders. Before purchasing or developing solutions, businesses must examine their long-term goals. Let's take a look at some of the benefits and drawbacks of buying and building solutions.
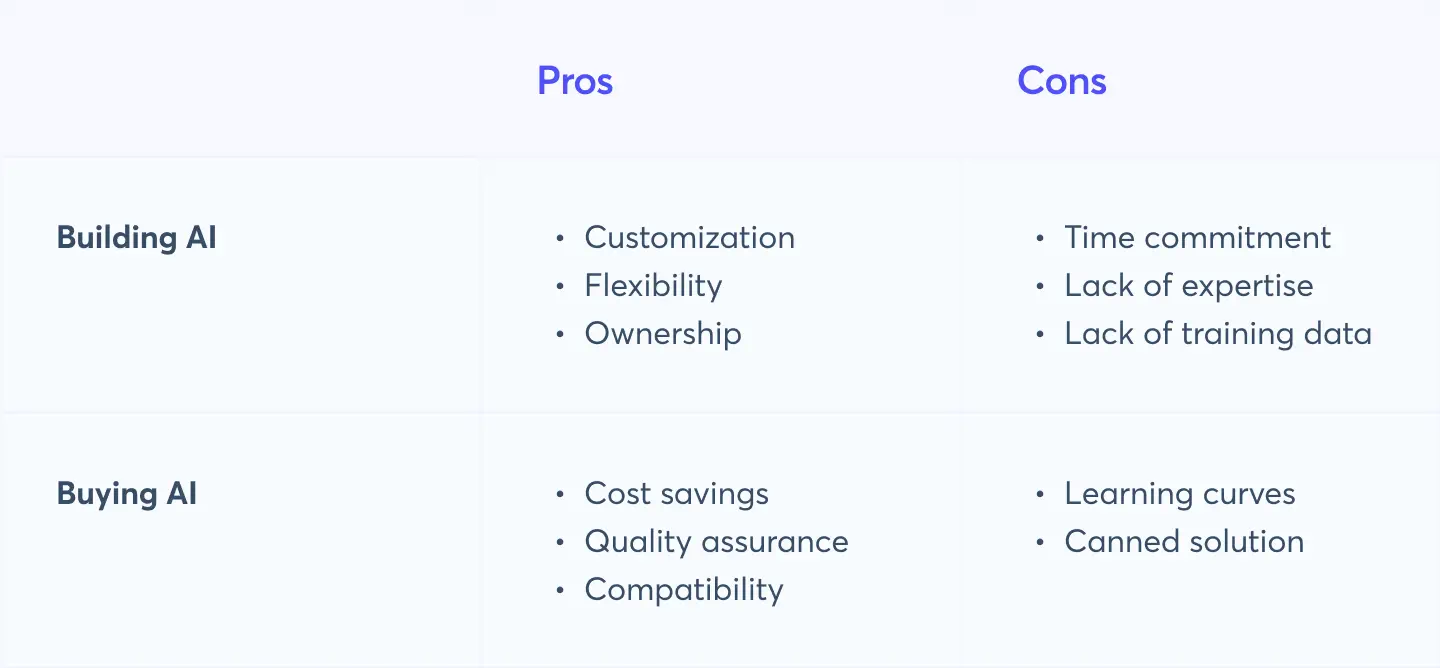
Recommended Reading: Solving the Build vs. But dilemma for ML
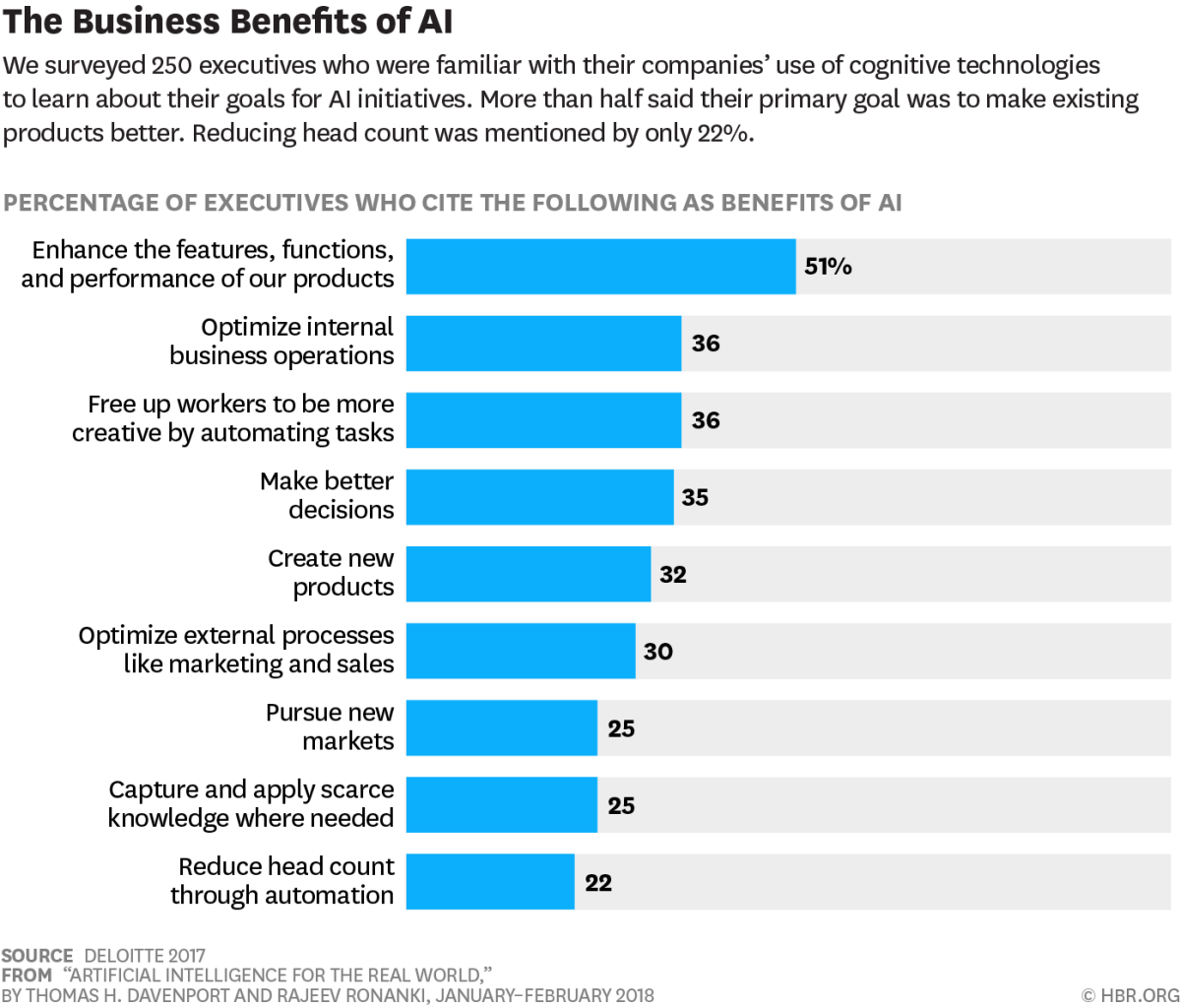
Open the Black box
As AI becomes more vital to mission-critical operations, technical teams implementing machine learning models and their executive counterparts must be completely in sync. By explaining model decisions, teams may create higher-performing and responsible models, boosting confidence among all stakeholders.
Explainable AI investigates why it was decided to make AI models more interpretable for human users, allowing them to comprehend why the system came to a certain conclusion or did a particular action. XAI contributes to AI transparency by allowing the black box to be opened and the whole decision-making process to be shown in a human-readable format.
- XAI helps bring down the cost of mistakes
- Improves confidence and trust
- Reduce the risk of model governance
- Increases performance of the model
Learn how Censius can help you in getting rid of the AI black box.
Explainability and Monitoring
Better tools are needed to monitor and debug ML model performance in the real world, and to assist teams in resolving issues before they impact business results. You can keep track of changes in performance by using model monitoring. As a consequence, you can assess the model's performance. It also helps you understand how to debug effectively if something goes haywire.
If you work in the banking or healthcare industries, monitoring a model’s health becomes very vital. With Censius’s automated monitoring feature, you can continuously monitor model inputs & outputs. To make your machine learning model effective, you should keep an eye on the following:
- Shifts in Data Distribution
- Changes in Performance
- Health Metrics
- Bias
- Data Integrity
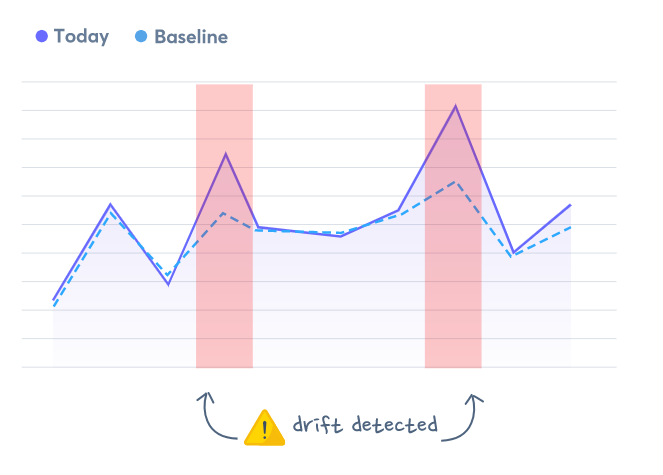
Start monitoring models
Conclusion
It's time for AI to become a greater part of the discourse as firms emphasize long-term value development. Enterprise AI companies may go a long way toward developing robust AI efforts that benefit all stakeholders by following a few easy measures. In this article, we saw how a sustainable approach could help AI and machine learning for business. I hope you liked this article.

Explore how Censius helps you monitor, analyze and explain your ML models
Explore Platform