5 things I wish I ‘caught’ as a data scientist working with LLMs
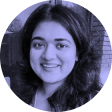
Censius
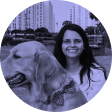
Key Takeaways
- 1
Uncover key gaps and challenges of Large Language Models in production
- 2
Understand the long-term implications of LLM issues such as hallucinations, bias, and drifts
- 3
Strategize ways to monitor LLMs in real-time to build reliable and high-performance models
Abstract
With the overwhelming capabilities and nuances of Large Language Models (LLMs), there is still a lot to overcome when it comes to navigating the intricacies of these huge models.
According to the Stanford University Artificial Intelligence Index Report (2022), a recent 280 billion-parameter model exhibited a substantial 29% increase in toxicity levels compared to a 117 million-parameter model from 2018. From handling model issues, hallucinations, biases, drifts, ethical considerations, and model underperformance, AI teams need to better strategize and foster more efficient workflows and build reliable LLMs.
Join a session with Censius experts where we deep dive into the hidden pitfalls encountered in LLMs at production; and how data science teams can prepare for the unexpected in the rapidly evolving field of building LLMs.